
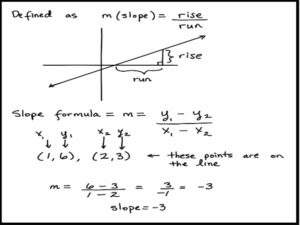
Linear regression is defined as a linear relationship between the response variable and predictor variables.
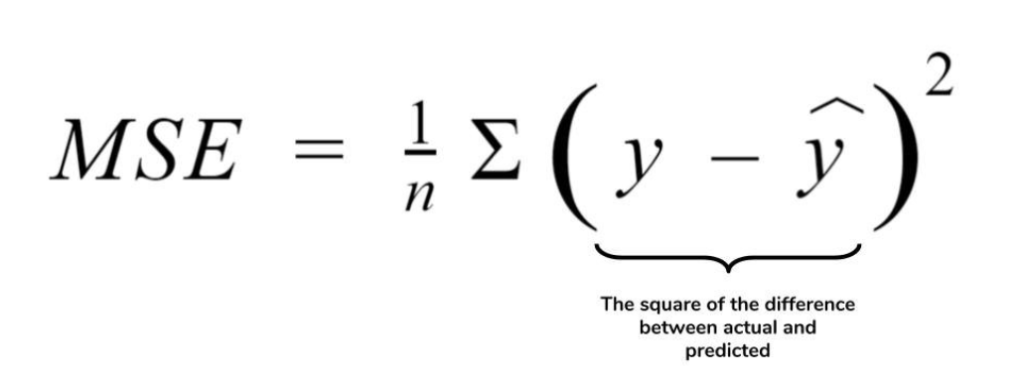
A nice feature of non-linear regression in an applied context is that the estimated parameters have a clear interpretation (Vmax in a Michaelis-Menten model is the maximum rate) which would be harder to get using linear models on transformed data for example. The most basic way to estimate such parameters is to use a non-linear least squares approach (function nls in R) which basically approximate the non-linear function using a linear one and iteratively try to find the best parameter values ( wiki). In non-linear regression the analyst specify a function with a set of parameters to fit to the data. As you may have guessed from the title, this post will be dedicated to the third option. In this case one may follow three different ways: (i) try to linearize the relationship by transforming the data, (ii) fit polynomial or complex spline models to the data or (iii) fit non-linear functions to the data. It is sometime fitting well to the data, but in some (many) situations, the relationships between variables are not linear. Drawing a line through a cloud of point (ie doing a linear regression) is the most basic analysis one may do.
